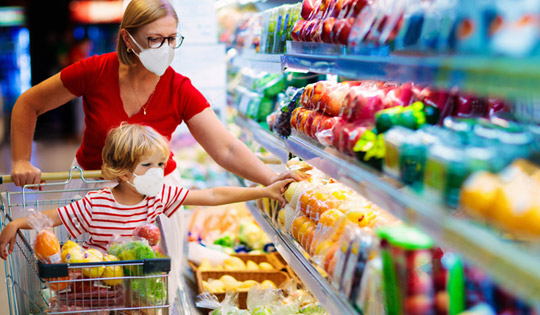
Objective
A top retailer wanted to increase sales for in-house brands as these brands drove higher profitability for the retailer. The goal was to understand each customer by creating behavioural cohorts and assess the propensity of each customer in a cohort to buy in-house brands.
Our Solution
- Our propensity computation module used a multi-model ensemble approach that included Logistic Regression, SVM, Boosted Decision Trees, Averaged Perceptron to identify in-house brand purchasing propensity for active customers.
- Our customer segmentation module created customer micro-segments (cohorts) based on customer behavior and attributes, taking into consideration demographic data, shopping mission, average monthly spend, sentiments and feedback, basket preference, average time between visits, bill value as well as baskets.
- The customer micro-segments were used to devise intervention strategies (such as personalized campaigns) for customers in each target cohort.
- Propensity models were used with a feedback loop that strengthened or weakened propensity scores with each transaction within an observation timeframe.
The solution was built leveraging tcg mcube, our proprietary analytics platform that can analyse large volumes of data to drive accelerated insights. The built-in data models and data science algorithms drive velocity to value for our customers.
Project Impact and Outcomes
Rollout of in-house brand related campaigns based on data science driven insights helped increase sales of in-house brands by over 15% within 3 months and this resulted in an overall profitability increase of approx. 5%.