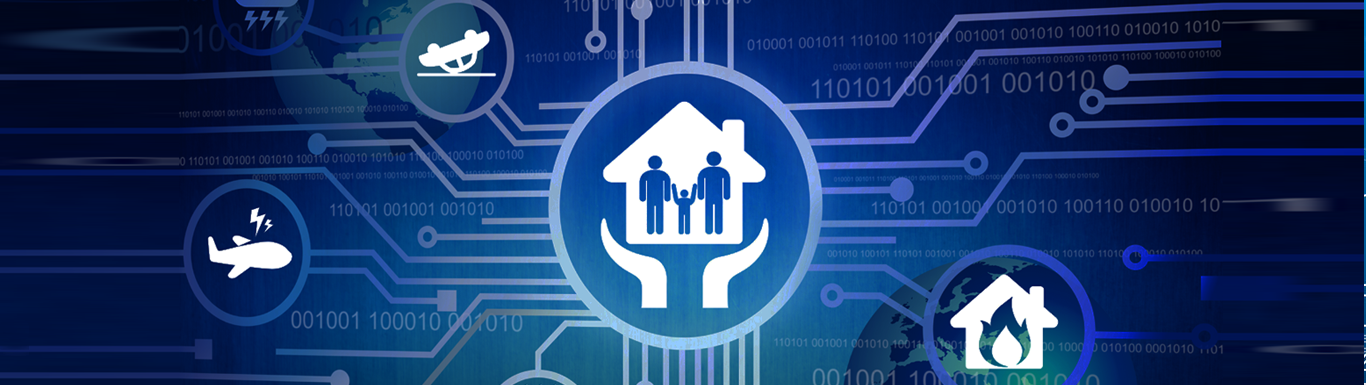
Did you realize that whether you are riding the Uber, instructing Alexa, wearing fitness trackers or using the smartphone, a vast amount of data is being generated? Interestingly, an estimate by experts reveals that by 2025, the volume of digital data will increase to 163 zettabytes. Today, this data acts as a treasure trove for most companies who consider evaluating it to generate actionable insights. This trend is sector-agnostic, and across domains, we see a similar pattern – be it in manufacturing, life science and healthcare, energy, retail, utilities or BFSI. The banking and financial services industry is one domain where data generated and handled is significantly enormous. As the electronic records only grow in number the financial services industry is turning to big data analytics to store data, generate actionable insights, and boost scalability. The insurers are not lagging behind, where they have access to rich sources of data and have figured out the right blend of what, why, and how to use the data to thrive in a highly competitive business environment.
A quick look at the challenges which plague today’s insurance companies tells us why harnessing customer data is more than important now. Growing risks, high operational costs, a volatile market, customer renewal, and churn remain major concerns. Data analytics, a significant part of data science helps address these challenges and facilitates claims processing, pricing strategies, and fraud management. Let’s look at some of these use cases in detail:
Risk assessment
Identifying risks and taking steps to mitigate them is a critical responsibility of any insurer, which becomes even more important when underwriting policies. There are cases where policies are written for storefronts and warehouses. In such cases, fixed data sets which include political, geographic, and economic data are analyzed. Furthermore, personal data of customers often prove to be an asset which when efficiently used helps analyze both types of risk— pure and speculative. The matrix model needs mention here which supports accurate risk assessment and facilitates meaningful risk discussions. In this 3×3 model, the event consequences lie along one axis and the event frequency on the other. Each group in the matrix represents risks at some level. The model is dependent on algorithms that detect and combine the data revolving around individual risks which vary in nature, effect, and character. This helps list each risk, rate its likelihood and severity and identify the highest-priority risks which may require immediate remediation.
Claims management
Apart from risk management, claims assessment is another important area where analytics plays a critical role. It is a given that faster and better insights empower insurers to ascertain and interpret what is going on in the claims process. However, data needs to be combined with analytics expertise to not only improve the speed of identifying hidden correlations but also respond to potential challenges timely. Analytics, in particular, helps prioritize claims, settle straightforward claims, tag the complex claims for further inquiry, and reduce the cycle time. Let’s take a case in point. A particular insurer’s data revealed that the claim cycle is abnormally high only in a specific geography. When data was dissected further, it was discovered that claim time for a particular claim in that geography was almost twice, and this was the case in bodily injury claims. This led to the understanding that by addressing the root cause of the cycle time, the insurer could decrease the average cycle time. The use of analytics led the insurer to specific questions such as the number of adjusters available to address bodily injury claims, idle time among adjusters, and so on which when solved expedited the claim cycle time.
Fraud Management
While we already know by now how analytics contributes to risk assessment and claims management, it also helps detect frauds. Handling frauds manually have always proved costly for insurers, more so when high-value frauds go undetected. Three innovative analytics methods in which frauds are detected include:
- Social Network Analysis (SNA)
In the case of SNA, both structured and unstructured data are fed into the ETL tool (Extract, Transform and Load tool). The analytics team then uses information across sources and calculates the likelihood of fraud based on several factors. Technologies that are integrated into the predictive modelling process for fraud management include text mining, content categorization, sentiment analysis along with social network analysis.
- Predictive analytics
The second method is predictive analytics, where text analytics and sentiment analysis are used to look at Big Data for fraud detection. Often the claim reports span across multiple pages leaving very little room for insurers to detect scams. Here analytics comes to use which not only helps sift through unstructured data but also detect possible frauds. Once the fraudulent claims are spotted, it accelerates the payment process of the legitimate claims leading to a higher number of satisfied customers.
- Social Customer Relationship Management (SCRM)
Social CRM or social customer relationship management is the third process in which the insurers link social media to the CRM. Insurers Invest in sophisticated analytics tools to verify the validity of claims and detect fraudulent crimes. This enables greater transparency with genuine customers and increases the faith they show in the insurance organization. Introducing social customer relationship management leads to a shift to a customer-centric ecosystem which is again beneficial to the business in the long run.
Final thoughts
As we reach the cusp of a massive data science revolution, the near future will witness insurance companies only increasingly using data science analytics. And why not when it helps optimize marketing strategies, enhance income, improve business and reduce costs. It will not be surprising if the use of data science in insurance makes huge leaps in the near future, and insurance businesses leverage it to the fullest. We may rather believe in this optimistic trend and wait and see what awaits us at the end of the road.